On February 18, 2021, NASA successfully landed its Perseverance rover on the surface of Mars after a 203-day journey to the Red Planet. Perseverance began its descent shortly after arriving, which required NASA to rely on the highly complex landing systems aboard the spacecraft to guide it to its final resting spot within Jezero Crater during a period known as the “seven minutes of terror.” Because the distance between Earth and Mars does not allow for real-time hand-steering of the rover to the surface, NASA utilized artificial intelligence (AI) to scan the landing site prior to landing the rover and safely guide Perseverance to its destination.
In addition to the landing process, NASA also equipped the rover with scientific instruments that use AI to perform guidance maneuvers and rock and sediment chemistry studies during its mission. The Planetary Instrument for X-ray Lithochemistry (PIXL) is attached to Perseverance’s robotic arm using a hexapod and is guided by AI to get the most accurate aim for its X-ray beam to determine rock textures and structures. The rover is also equipped with a SuperCam that uses AI to identify, zap, and vaporize rock targets with a pulsed laser to study their chemical makeup.1
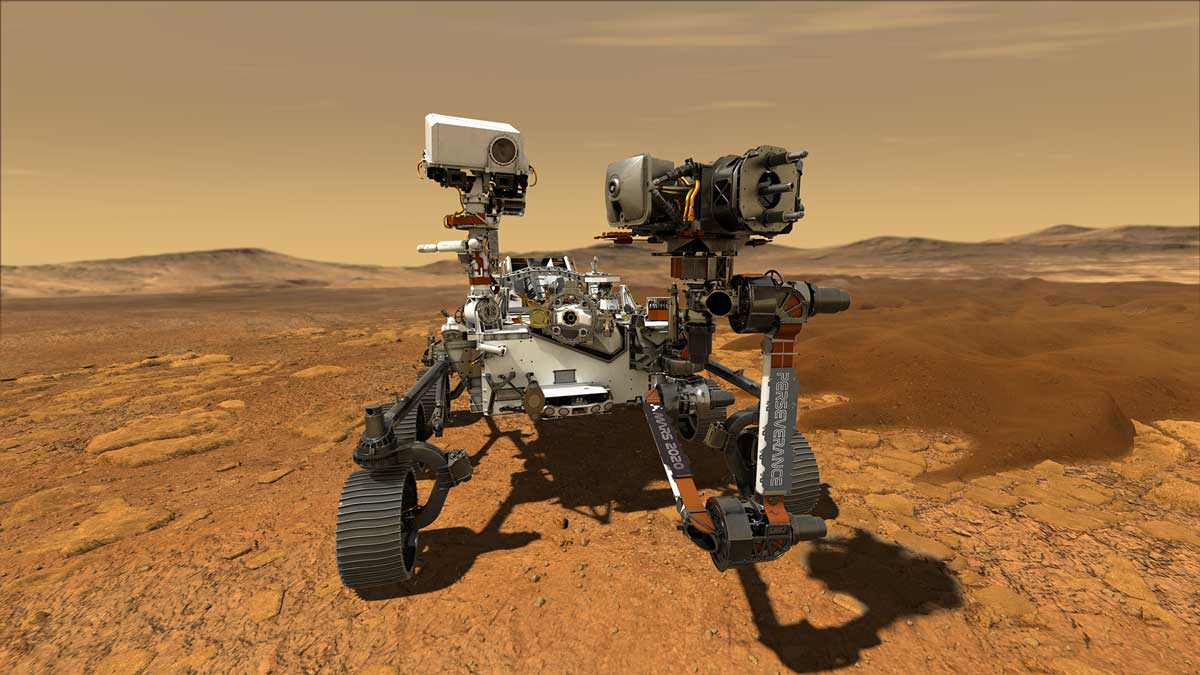
Figure 1: Artist Concept of the Perseverance Rover on Mars. Credit: NASA
What is AI & Machine Learning?
AI refers to the simulation of human intelligence in machines that are programmed to think like humans and mimic their actions.2 This also extends to any machine that perceives its environment, takes actions, and mimics cognitive functions associated with a human mind such as learning and problem-solving. AI aims to solve traditional problems like reasoning, knowledge representation, planning, learning, perception, and the ability to move and manipulate objects based on a given situation.3
One of the most commonly cited benefits of AI is automation, which has significant impacts across multiple industries. Automation is used to improve productivity and increase production and allows for more efficient use of materials and reduced lead times. AI in automation helps streamline resources to provide availability for more critical or complex tasks.
We use AI to make smarter decisions. With technology that can be used to coordinate data delivery, analyze trends, develop data consistency, provide forecasts, and quantify uncertainties, AI technology can help us make the best unbiased decisions for a specific scenario.4 AI systems eliminate human emotion, drawing patterns in data and delivering accurate results faster than human capabilities.
We also utilize AI and machine learning technology to understand the potential outcomes of different scenarios and trends. Machine learning algorithms build predictive models based on sample data to create patterns and can make conclusive decisions without being explicitly programmed to do so. We further use a form of machine learning, called deep learning, to mimic human capabilities of performing classification tasks directly from image, text, and sound data. We train these algorithms with big data and artificial neural network (ANN) architectures that contain many layers, which is particularly important for safety-critical applications.
We benefit from AI and machine learning by using these technologies to reduce human error, handle repetitive jobs, and maintain business continuity. Similarly, AI and machine learning technologies positively impact our support to the complex missions that define the future of exploration and national security operations in space.
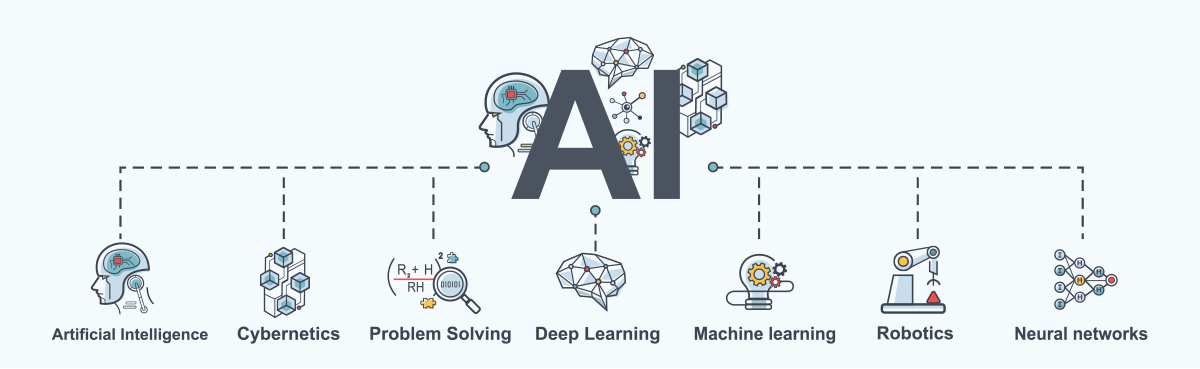
Figure 2: Artificial Intelligence and Machine Learning
AI & Machine Learning in Space Operations
Current space exploration missions use AI and machine learning capabilities in many areas of space operations including mission planning and operations, data collection, autonomous navigation and maneuvering, and spacecraft maintenance. Future missions will need to rely on the same.
Mission Planning
The Entry, Descent and Landing (EDL) flight dynamics team had to rely heavily on AI for mission planning and complex scheduling systems to get through the “seven minutes of terror” during the Perseverance mission. Mission scientists and engineers consider scheduling to be a domain where we can apply AI techniques due to the intricate planning involved with these systems. Without the use of AI, scheduling for a mission requires large teams to work many hours to meet the requirements of the mission. By using AI, we can alleviate the need for these human resources by programming the spacecraft to determine for itself how to intelligently execute a command given a specific function based on past experiences and its environment.5
Realistic scheduling problems typically involve many constraints. Offsetting conflicting constraints and performing trade-offs greatly increases the complexity of scheduling. We can use AI to address key scientific and engineering mission constraints and optimize the schedule accordingly to satisfy all needs.
Mission Operations
AI is used for autonomous operations for space missions. Through the European Space Agency’s (ESA) technology transfer program, the Italian start-up company, AIKO, developed a software library called MiRAGE that is used to enable autonomous operations for space missions. Using these operations, the spacecraft itself can perform autonomous replanning, detect events (both internal and external), and react accordingly, ensuring fulfillment of mission objectives without the delays introduced by the decision-making process on the ground.6
We can also use AI and machine learning to assess safety-critical missions and evaluate operational risk analysis. These technologies allow for risk mitigation systems to process large amounts of information based on indicators from nominal operations and past performance where anomalies occurred. However, without deep learning using ANNs, we could not confidently assign a risk level to events and would require expert supervision as a result. Once we have trained a model to identify risk categorization, it can use the knowledge to evaluate real-time risk assessments.
Data Collection
Over the past decade, the volume of data we have collected from Earth-observing spacecraft, deep space probes, and planetary rovers has increased significantly for ground infrastructures used to distribute and deliver the data to multiple end users. Having the ability to optimize the vast amount of data collected from scientific missions and analyze the data using AI automation positively impacts how data is processed and transmitted to the end user.
AI used aboard spacecraft can autonomously detect and characterize features that are nominal, such as typical weather patterns, and differentiate them from unusual patterns like smoke plumes from volcanic activity to create maps and data sets. We can use AI to determine which data sets are important to send to ground segments for processing. We can also use AI technologies to remove data that provides little to no significance. This can ease burdens or constraints the space-to-ground networks experience with the transmission of large-volume data.
Autonomous Navigation & Maneuvering
As we saw during Perseverance’s arrival to Mars, ground operation communication capabilities do not always allow for continuous human monitoring and operations of the spacecraft. Autonomy in navigation allows for spacecraft to travel through interstellar space and perform complex maneuvers for arriving at and landing on distant celestial objects. Human intervention to control a spacecraft cannot occur in real-time from the ground due to the lag in communications in deep space. Since spacecraft cannot wait for commands in given situations that require immediate determinations, using AI and machine learning facilitates guiding and maneuvering a spacecraft while freeing up human resources for other mission activities.
The risk of collisions between man-made objects in space is growing. With the increase in space debris and the launch of mega-constellations, the situation is expected to get worse. Therefore, another area where machine learning is being tested is in space situational awareness and conjunction events, where two or more spacecraft arrive at the same point and time. Ideally, by identifying conjunction events requiring maneuver action, we can capture specific data for events and operator responses to feed into a machine learning algorithm in order to determine and mitigate future events. However, edge-case scenarios or scenarios that occur only at an extreme operating parameter, can be difficult for training since they can be unique and hard to capture.
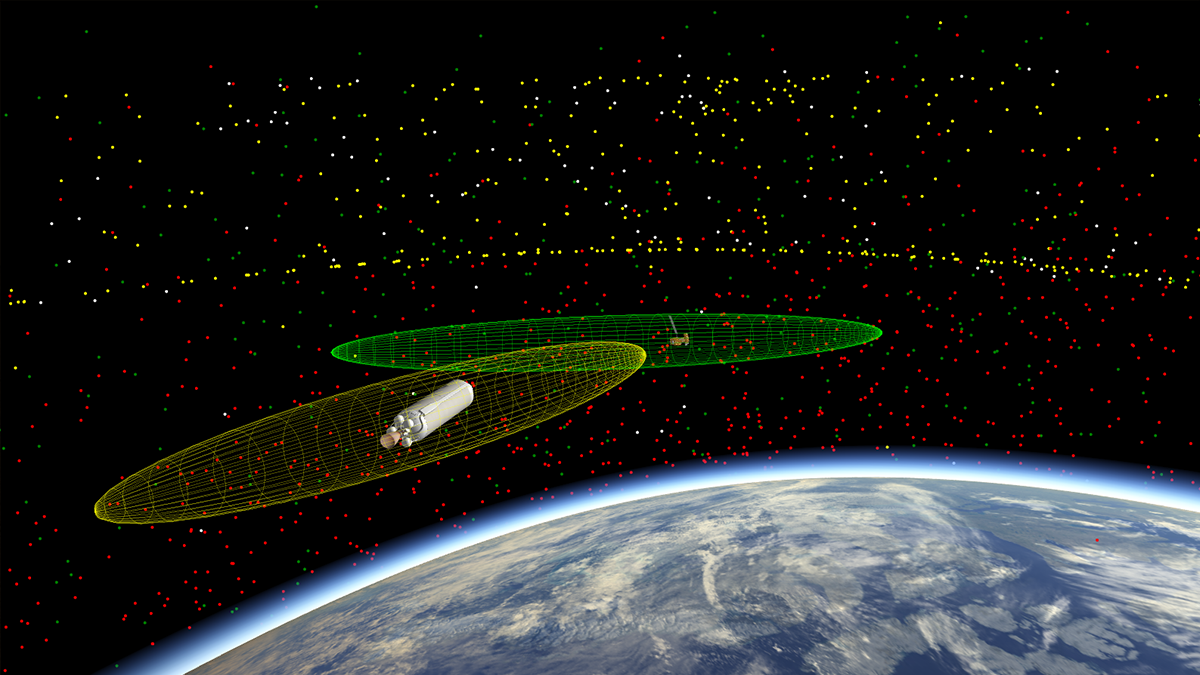
Figure 3: Spacecraft Conjunction Simulation Using FreeFlyer Software.
On-Orbit Spacecraft Maintenance
As we investigate the future of space exploration, we cannot overlook the need for spacecraft maintenance missions. The spacecraft we are launching today are designed for routine assisted and self-maintenance including hardware upgrades, refueling, and service for jammed systems like solar arrays.
NASA’s On-orbit Servicing, Assembly, and Manufacturing 1 (OSAM-1), formerly called Restore-L, is a robotic spacecraft equipped with the tools, technologies, and techniques needed to extend the life of satellites, even if they were not designed for servicing. During its mission, the OSAM-1 spacecraft will rendezvous, grasp, refuel, and relocate a U.S. Government-owned satellite to extend its life. Using the attached payload called Space Infrastructure Dexterous Robot (SPIDER), OSAM-1 can assemble and install a 9-foot communications antenna and add a 32-foot beam to the government satellite.7
The maintenance mission technology supported by AI and machine learning technologies includes autonomous, real-time relative navigation systems, dexterous robotic arms that precisely execute assignments, and advanced tools to execute servicing tasks.
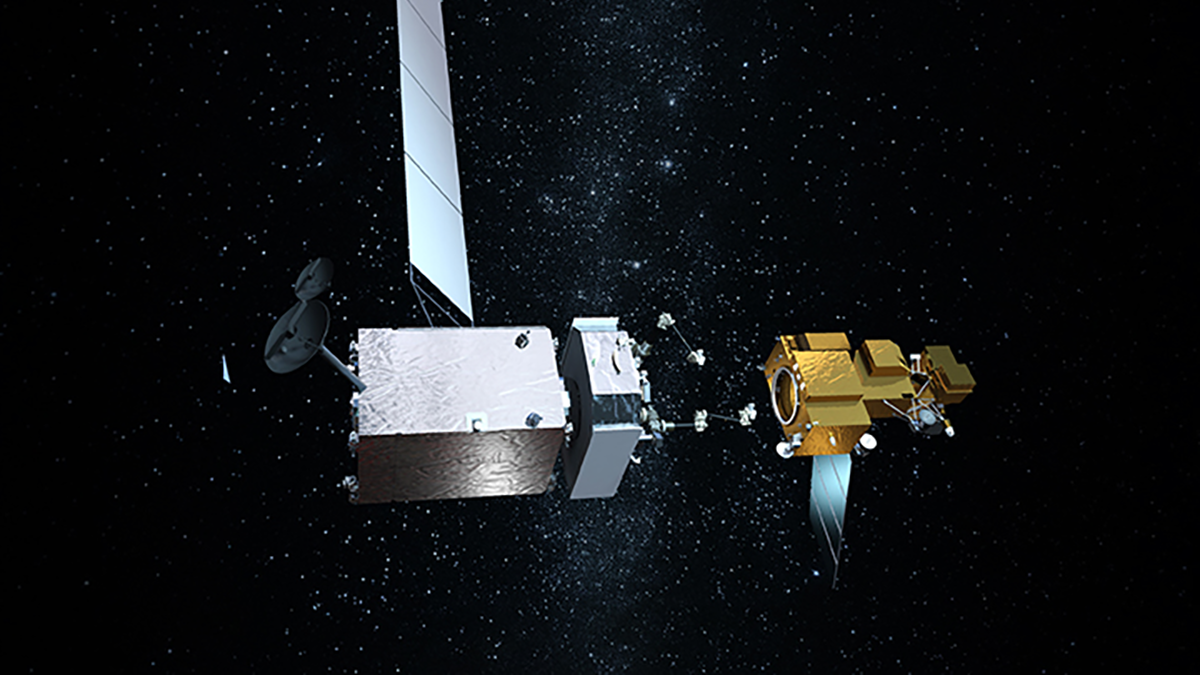
Figure 4: Artist Concept of the OSAM-1 Servicing Mission. Credit: NASA
Conclusion
AI and machine learning capabilities are making significant impacts in the space industry by creating efficiencies in mission planning and operations and providing scientists with the ability to explore the far reaches of space. While automation of tasks paves the way for the use of AI, the ability for spacecraft to become fully cognitive machines, capable of making critical decisions based on their current environment, without reliance on ground systems to perform essential functions will create more time for humans to spend on valued and more complex research activities.
Sources
- NASA – Mars Perseverance Press Kit: https://www.jpl.nasa.gov/news/press_kits/mars_2020/download/mars_2020_landing_press_kit.pdf
- Investopedia – Artificial Intelligence (AI): https://www.investopedia.com/terms/a/artificial-intelligence-ai.asp
- Wikipedia – Artificial Intelligence: https://en.wikipedia.org/wiki/Artificial_intelligence
- 10XDS – Top 10 Benefits of Artificial Intelligence (AI): https://www.10xds.com/blog/benefits-of-artificial-intelligence-ai/
- Medium.com – Artificial Intelligence for Space Exploration: https://medium.com/@apltownsend/artificial-intelligence-for-space-exploration-703d2838e37c
- ESA – AIKO: Autonomous Satellite Operations Thanks to Artificial Intelligence https://www.esa.int/Applications/Telecommunications_Integrated_Applications/Technology_Transfer/AIKO_Autonomous_satellite_operations_thanks_to_Artificial_Intelligence
- Goddard Space Flight Center – NExIS OSAM-1: https://nexis.gsfc.nasa.gov/osam-1.html